Turner-Fairbank Highway Research Center
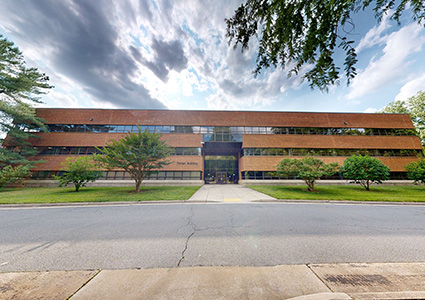
With 15 cutting-edge laboratories onsite, Federal Highway Administration’s Turner-Fairbank Highway Research Center is the Nation’s premier facility for advanced and applied research and development related to new and existing highway technologies.
Highway Safety Information System Student Contest
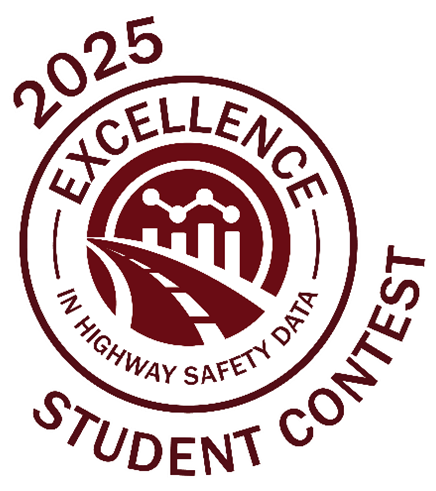
Win money and prizes for original safety research! The Excellence in Highway Safety Data Award encourages undergraduate and graduate students to use roadway data to research a safety topic and prepare for a career in highway safety. Learn more…
You can request HSIS data here: https://securedatacommons.atlassian.net/servicedesk/customer/portal/6.