The Development of Data Fusion and Visualization Tools for Nondestructive Evaluation of Bridges
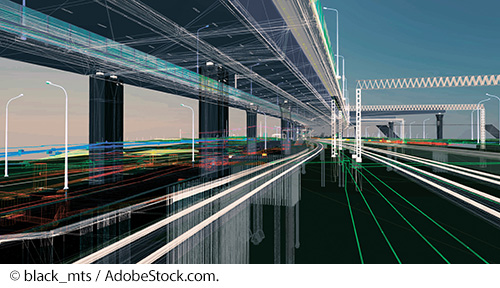
The Nation’s highway infrastructure requires condition assessment of more than 620,000 bridges to ensure safety, sustainability, and resiliency. Traditional methods of assessing bridges, such as coring or chain dragging, can cause economic and time constraints as well as pose safety risks to the structure’s integrity. However, revolutionary techniques are changing the way assessments are conducted and how bridge engineers are looking to a safer future—without physically impacting structures.
Nondestructive evaluation (NDE) of bridges involves the assessment of various structural components using acoustic, electrochemical, and/or electromagnetic methods, which do not change the structure’s physical properties or structural integrity. Based on the latest data from InfoBridge (https://infobridge.fhwa.dot.gov/), more than 42,000 bridges in the Nation have been classified as poor condition, representing nearly 217 million square feet of deck area that needs prominent inspections. The implementation of NDE technologies, combined with the latest advancements in artificial intelligence (AI), hold the potential to significantly enhance the accuracy, efficiency, and economic viability of condition assessments of bridges. These technologies can play a crucial role in ensuring the ongoing structural integrity of aging bridges.
Data fusion is widely applicable across various fields and industries. In the context NDE, data fusion combines data from disparate sources to produce a probabilistically sound estimate of an object’s current state. Recent advancements in the field of NDE have led to the use of multiple evaluation modalities, and fusion of these modalities can provide a more reliable condition assessment by leveraging the complementary strengths of different NDE technologies. Data fusion is expected to produce high-quality and definitive results that can assist in providing a better understanding of a bridge’s condition.
In 2020, the Federal Highway Administration’s (FHWA) NDE Laboratory launched a research project focusing on the development of data fusion and visualization tools for the NDE of bridges and highway infrastructure. This project, currently in progress at the lab at the Turner-Fairbank Highway Research Center, aims to develop user-friendly data fusion and visualization tools for bridge inspectors to enhance current bridge inspection practices.
These tools streamline analysis and fusion of multiple NDE sources by using cutting-edge techniques in machine learning, deep learning, and AI. The goal of these tools is to increase confidence in detecting, diagnosing, and quantifying bridge defects using various NDE technologies, including impact echo (IE), ground penetrating radar (GPR), ultrasonic surface waves (USW), half-cell potential (HCP), and electrical resistivity (ER), among others.
“Improving the ability to visualize and fuse multiple NDE data modalities provides valuable insights into the condition of bridge decks. It can potentially lead to better decisionmaking, more targeted maintenance strategies, and subsequent safety and serviceability of bridges,” says Jean Nehme, director of FHWA’s Office of Infrastructure Research and Development.
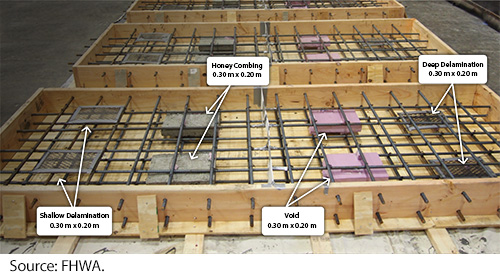
Laboratory Specimen for Concrete Bridge Decks
Given that bridge decks are statistically prone to faster deterioration than other bridge components, concrete bridge decks represent an area of high priority with the research and development of NDE technologies.
The FHWA project has used data acquired from laboratory testing of eight controlled specimens with various artificial defects, such as delaminations, honeycombing, voids, vertical cracks, and precorroded reinforcing steel. These specimens were then tested by various NDE technologies, including manual sounding, IE, USW, ultrasonic shear-wave tomography, infrared thermography, GPR, ER, HCP, and impulse response. Embedded artificial defects provide a known ground truth (e.g., types and/or locations of defects) for validating the NDE results and labeling the collected NDE data. The labeled data is a valuable resource in the supervised training of AI models. The well-trained models can analyze raw NDE data as inputs with automated damage detection and localization as outputs. Testing these controlled specimens has generated numerous AI models for automated NDE data analysis, including IE and GPR. These AI models built the basis for the following data fusion and visualization.
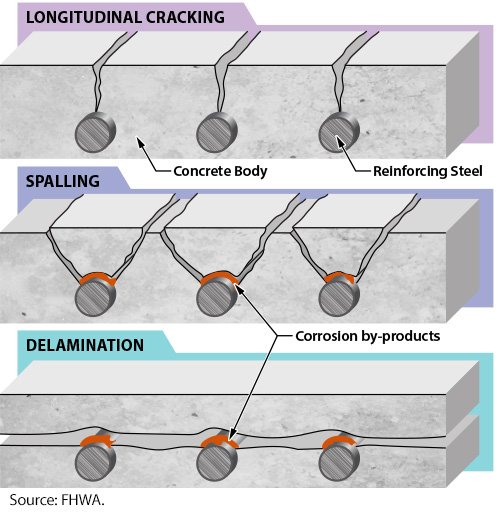
Data Fusion and Visualization
Data fusion integrates the strengths of multiple NDE modalities to provide an effective pathway for quantitative and spatial cross-validation of assessment results. One example of this integration uses IE, GPR, HCP, and ER for assessing shallow delamination, which typically occurs in the early stages of deck deterioration when corroded rebar expands and results in horizontal cracking at that rebar layer. If the deck continues to deteriorate, the horizontal cracking may expand further and form a delamination that may eventually spall.
The delamination underneath the deck surface changes the mechanical properties of the deck locally. As a stress-wave-based NDE technique, IE is particularly sensitive to this change and detects delamination by observing shifts in the peak frequency of collected wave signals. However, because concrete is a heterogeneous material, local changes in material properties may also introduce slight shifts in peak frequencies. While IE is a reliable method for identifying delaminations in a bridge deck, it does not provide any information about the potential cause of the delamination.
GPR is typically used to assess the cover depth of rebar in concrete decks or to identify precursors to delaminations such as chlorides, moisture, or potential cross-section loss of the reinforcing steel. GPR emits electromagnetic (EM) waves into bridge decks and collects reflected signals. The transmission inside the material and reflection at material interfaces of EM waves depend on that material’s dielectric constant or the relative permeability. The reflection of EM waves occurs in the presence of dielectric contrasts; the higher the dielectric contrast at the interface, the more energy of EM waves will be reflected. In the case of a bridge deck, the dielectric constant of concrete material is around 8, while the dielectric constant of the reinforcing steel is theoretically infinite. The infinite dielectric contrast between the steel and concrete results in a nearly perfect reflection of energy when EM waves reach to reinforcing steel in concrete. On the other hand, water has a dielectric constant of around 81, leading to a smaller reflection than the one from steel. Therefore, low signal amplitudes near reinforcing steels are typically associated with the presence of water, chlorides, or loss of steel cross-section, which are precursors to a delaminated area.
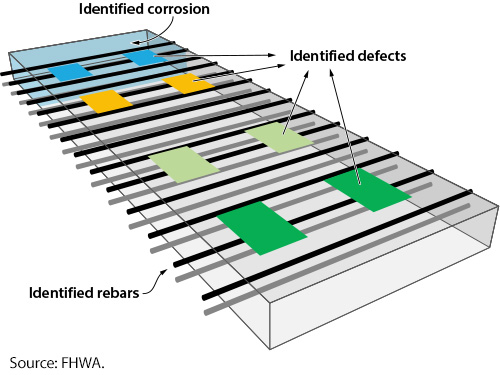
Electrochemical methods such as HCP and ER are common technologies used for corrosion assessment and are typically associated with the measurement of the probability of corrosion of the top mat of reinforcing steel. The combination of GPR with HCP and ER would provide complementary information to determine if the degradation identified by GPR is also presenting active corrosion. Active corrosion in the reinforcing steel of concrete bridge decks can better inform bridge owners about proper asset management with rising priorities.
While each NDE technology provides a measurement within a specific portion of the life cycle of a concrete bridge deck as it relates to corrosion-induced degradation, the fusion of the datasets can lead to a better understanding of the cause of degradation and subsequently provide asset owners with more information for data-driven decisionmaking.
Using the datasets collected on the fabricated decks during the research project, a three-dimensional reconstruction of the deck’s deterioration can be achieved by fusing the knowledge gained from these NDE modalities. The results closely match the ground truth, with reinforcing steel identified at the top layer beneath the concrete cover. Cross-validation of IE, ER, and GPR results confirms the delamination in the corroded area near the top layer of reinforcing steel.
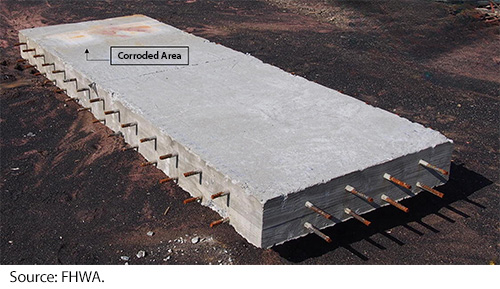
Next Steps
The success of data fusion relies on the successful acquisition and accurate analysis of each complementary NDE technology. Integrating current NDE technologies with machine learning and AI is one of the future goals of this FHWA project. The integration aims to provide more robust assessments for each technology and pave the road for advanced data fusion. Collecting more data and developing advanced algorithms for data analysis is another future effort designed to improve trained models for degradation prediction based on single NDE datasets. Those research results will be shared with Federal, State, local, academic, and industrial partners to foster NDE technology development and transfer.
“The integration of various NDE data sources and AI enables us to make more accurate predictions, identify potential problems earlier, and develop more efficient maintenance strategies,” says Anne Rearick, director of bridge management for the Indiana Department of Transportation. “This approach has the potential to greatly enhance the safety and reliability of bridges while reducing costs and minimizing disruptions to traffic flow. Ultimately, the integration of these technologies has the potential to revolutionize the way we approach bridge deck assessment and maintenance, leading to a more sustainable and resilient infrastructure system.”
Hoda Azari is the manager of the NDE research program and NDE Laboratory. She holds a Ph.D. in civil engineering from the University of Texas at El Paso.
Heng Liu is a contracted research engineer working in the NDE Laboratory. He holds a Ph.D. in civil engineering from the University of Maryland, College Park.
Rahul Torlapati is a contracted data analyst working in the NDE Laboratory. He holds an M.S. in data science from the University of Maryland.
For more information, see https://highways.dot.gov/research/laboratories/nondestructive-evaluation-laboratory/ongoing-projects, or contact Hoda Azari, 202–493–3064, Hoda.Azari@dot.gov.